It used to take hours to create a high-quality podcast. NotebookLM can do it in around 5 minutes with eerily accurate and conversational results.
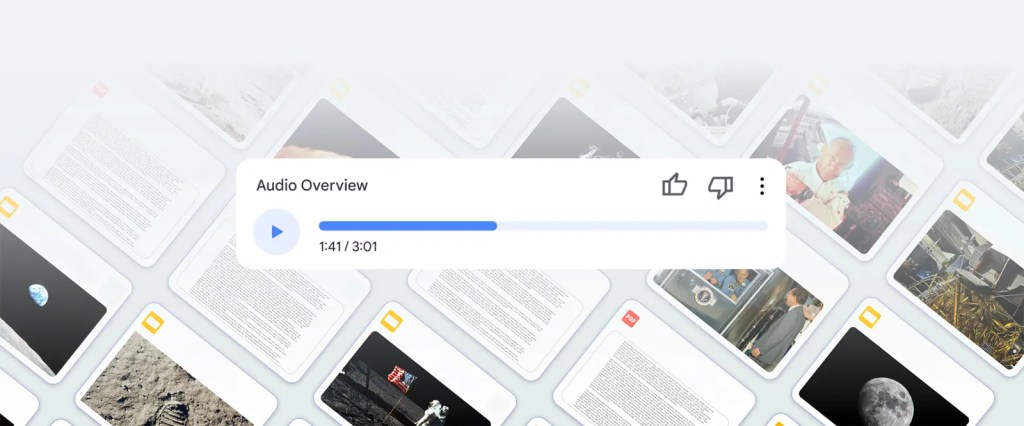
There is a saying in computer science that “garbage in equals garbage out”. Over the last two years, the rise of large language models (LLMs) has brought that expression to the forefront of public awareness. Feeding garbage into an AI model can result in garbage – or nonsense and hallucinations – coming out of it.
Google’s new product, Notebook Language Model (NotebookLM), seeks to remedy nonsensical output, by ‘training’ on precise, targeted information, rather than data from a large broad source such as what can be scraped from the internet. That output can be seamlessly and quickly made into a high-quality podcast.
Biao Wang is a NotebookLM product manager based at Google Labs in Mountain View, California.
“With one click, two AI hosts start up a lively “deep dive” discussion based on your sources. They summarise your material, make connections between topics, and banter back and forth,” says Wang.
‘Notebook’ is a product that was designed in the U.S., but like many tech innovations, quickly rolled out across the world. Google Australia’s managing director Melanie Silva says she has been using the audio feature to listen to long briefing documents sent to her in text format.
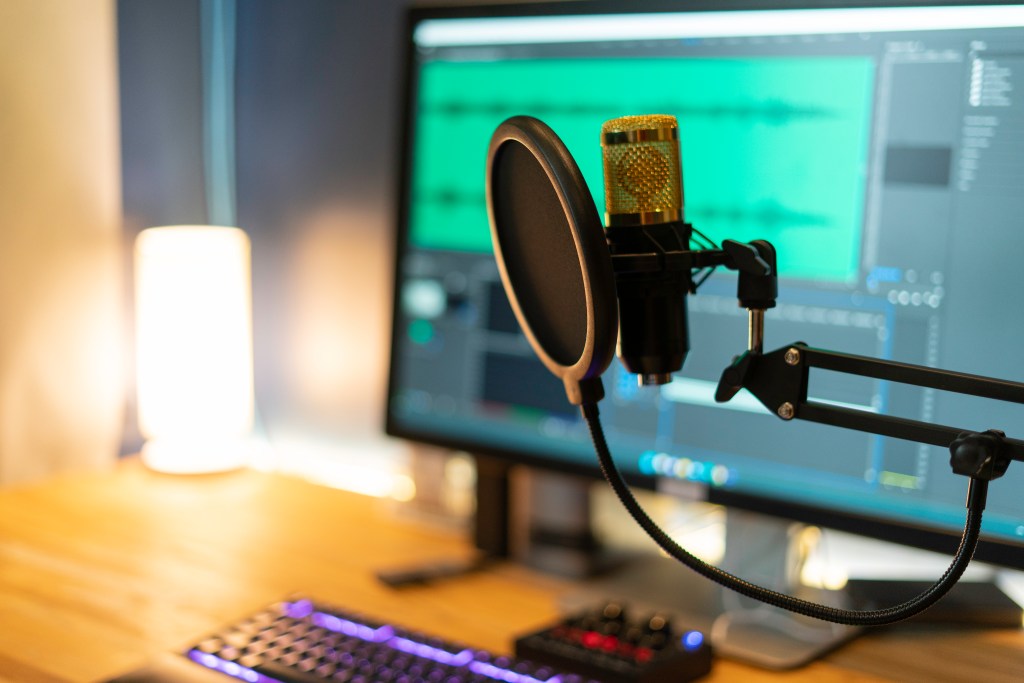
“It’s basically like having your own personal large language model or LLM – a super-smart research assistant that can read your documents, summarise them, answer your questions, quiz you, and even help you brainstorm new ideas,” says Silva.
The release of this product comes at a time when podcasting is accelerating at a rapid pace. The Infinite Dial released research in April that showed 20% more Aussies listen to podcasts than two years ago. Forty-eight per cent of our population aged 12 years and older listened to podcasts in the last month, up from 43% in 2023.
Testing the podcast waters
I put NotebookLM to the test by uploading six of my recent Forbes Australia articles and asking it to create an audio file. Less than a minute later I had an accurate 250 word synopsis of the six articles. Three minutes after that my downloads folder contained a 7-minute fully-produced ‘podcast’ featuring two AI voices discussing the ‘news of the week’ using data and quotes from the articles I had uploaded.
The audio production quality is alarmingly good.
The AI hosts speak in a conversational tone with pauses, cadence, and inflections appropriate to the context. The audio sounds human, a marked improvement from yesteryear’s staccato ‘computer-generated’ voices.
Given the quality and speed in which the audio is generated, the time and cost that NotebookLM can save podcast content creators cannot be underestimated. No editor, sound engineer, or host was paid to create the podcast created from my articles. Typically, anything AI-generated is cause for suspicion about accuracy, but this audio is derived exclusively from stories I have factchecked – ultimately giving me more confidence in its efficacy.
Repurposing content from one format to another raises an important question about intellectual property – does ownership and access to the source material change once it is uploaded to the LM, and, who ‘owns’ the output that is generated from it? In the first instance, Google has been clear – nothing that is uploaded to NotebookLM becomes its property. On the second count, I asked Google and have not received an answer.
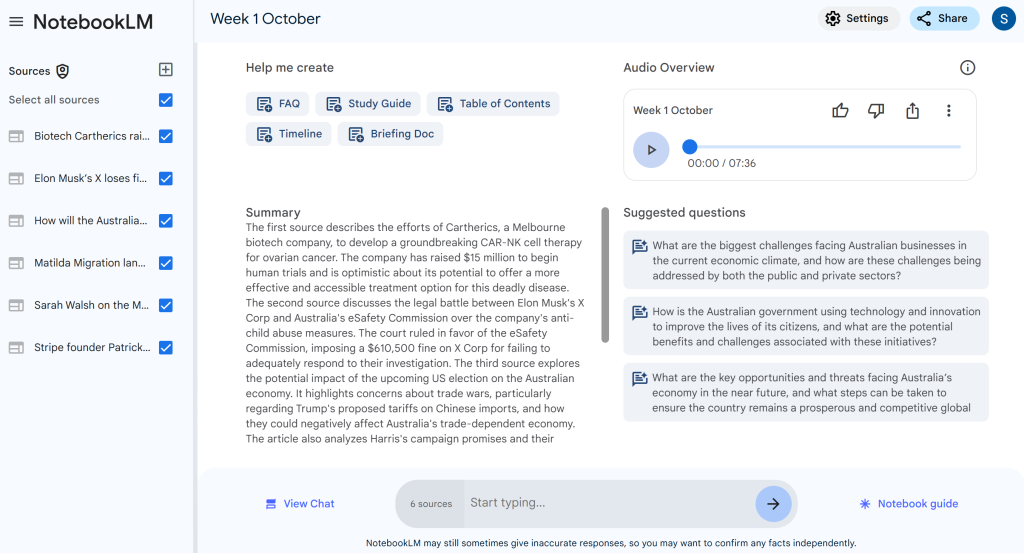
As with all AI-generated material, it is essential to have a ‘human in the loop’ to ensure the accuracy of the podcast conversation. Google notes as much on its webpage, warning in small font at the bottom of the page that ‘NotebookLM may still sometimes give inaccurate responses, so you may want to confirm any facts independently.’
Data privacy
Steven Johnson is the editorial lead for Google Labs. He notes that Input fed into NotebookLM is only used to create output in that session, and is not stored by Google to train other LLMs.
“We are putting your information into the short-term memory of the model. (Technically called the model’s ‘context window,’)” says Johnson.
“If you ask a question about, say, your company’s marketing budget for 2024 based on documents you’ve uploaded, NotebookLM will give you a grounded answer, but the second you move on from that conversation, the quotes are wiped from the models’ memory.”
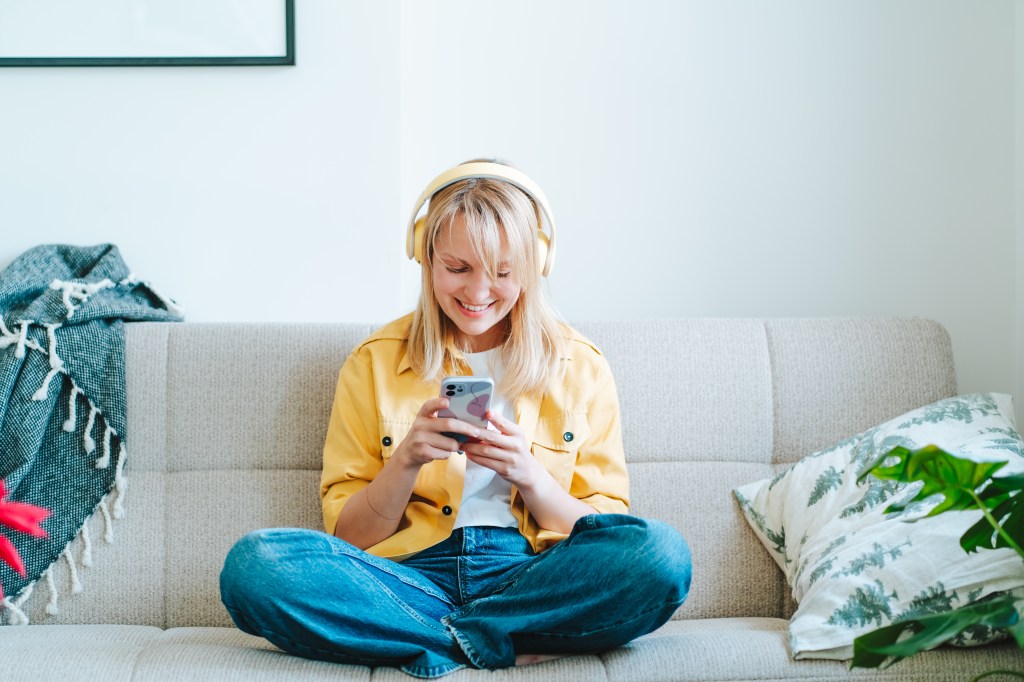
Johnson is a New York Times journalist and 12-time author who joined Google in 2022 to work on NotebookLM. He set out to create a solution to a problem that he faced as a writer – being able to collate, summarise, access, and repurpose vast amounts of research. Ensuring that the AI solution kept his work private was essential, Johnson says.
“You get all the power of summarisation and explanation that the language models give us, but at the same time you can feel secure that whatever personal information you share with NotebookLM will stay private if you choose,” says Johnson.
Avoiding hallucinations and inaccurate information is essential to the work Johnson and journalists all over the world do. ‘Small language models,’ developed from trusted sources, or a reporter’s notebook, are an ideal way forward.
“When you upload your sources, it instantly becomes an expert, grounding its responses in your material with citations and relevant quotes. And since it’s your notebook, your personal data is never used to train NotebookLM,” confirms product manager Wang.
Look back on the week that was with hand-picked articles from Australia and around the world. Sign up to the Forbes Australia newsletter here or become a member here.